Understanding Backtesting: Essential Tool for Investment Professionals
- Aki Kakko
- Jun 6, 2023
- 4 min read
Updated: Feb 17, 2024
Backtesting is a vital tool for investment professionals, providing a method to assess the efficacy of trading strategies, forecasting models, or financial algorithms. It is the process of applying a particular strategy or model to historical data to evaluate its performance during a specific period. It helps answer the question: "If I had implemented this strategy in the past, how well would it have performed?" This article will delve into the concept, advantages, limitations, and application of backtesting.
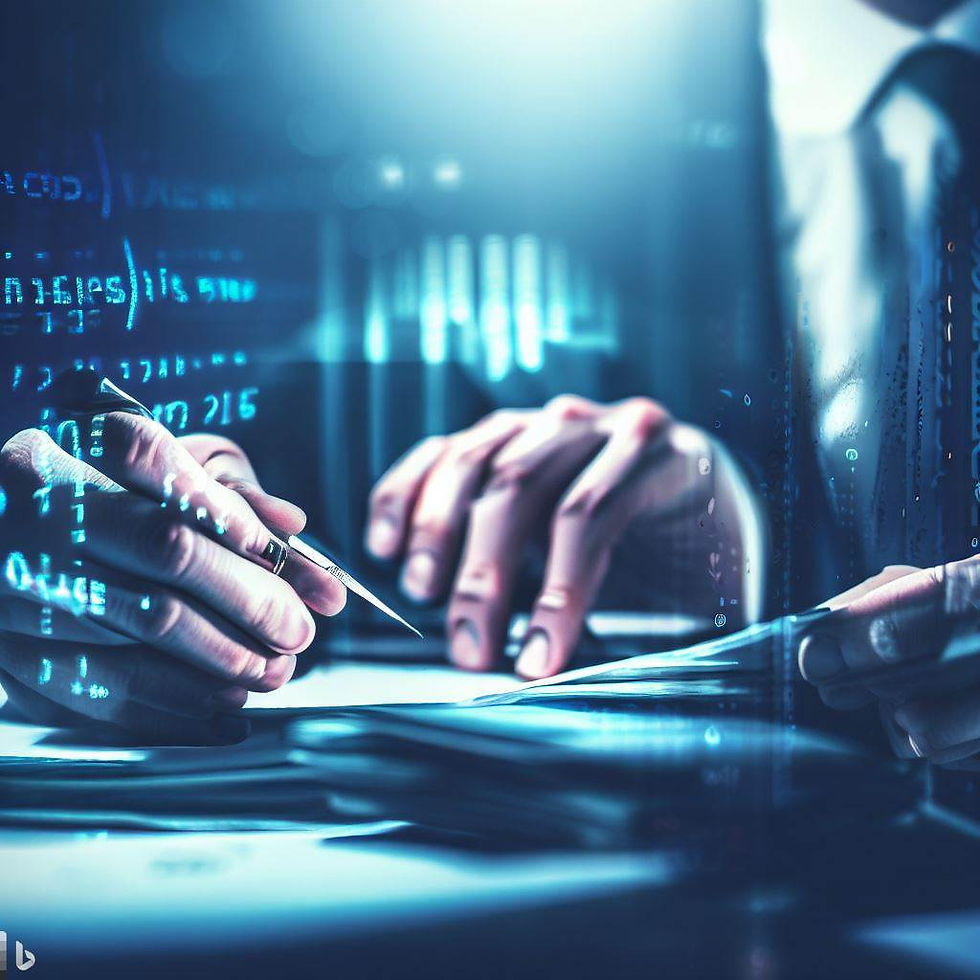
What is Backtesting?
In its most basic form, backtesting involves simulating a strategy on historical data and then analyzing the results. The strategy could involve stocks, forex, futures, options, or any other financial instrument. By using past data, we can get an estimate of how well a strategy would have performed had it been implemented during that time. A simple example is a moving average crossover strategy in the stock market. This strategy triggers a buy signal when a short-term moving average (e.g., a 50-day moving average) crosses above a long-term moving average (e.g., a 200-day moving average). A sell signal occurs when the short-term moving average crosses below the long-term moving average. Backtesting this strategy would involve applying these rules to historical stock price data and calculating the hypothetical returns.
Advantages of Backtesting
Validation of Strategy: Backtesting allows traders to validate their trading strategies before implementing them in real time. A strategy that consistently performs well in backtests may have a good chance of success in the future.
Improvement of Strategy: Backtesting not only validates but also helps to refine and optimize strategies. Traders can adjust parameters and see how these changes affect performance.
Risk Management: Backtesting can provide statistics about potential drawdowns, helping traders manage risk more effectively. This can guide decisions on position sizing and risk tolerance.
Confidence Building: Positive backtesting results can increase a trader's confidence in their strategy, potentially leading to better decision-making and fewer instances of second-guessing.
Limitations of Backtesting
Overfitting: This occurs when a model is excessively tailored to fit historical data and may not perform well on new, unseen data. A backtest can easily be 'overfit' if it's overly complex or if it's based on a small set of data.
Data Snooping Bias: This is a form of bias that happens when a strategy is seemingly optimized for the dataset it was backtested on, but performs poorly on new data.
Market Regime Changes: Markets are dynamic and constantly changing. A strategy that worked well in the past may not work in the future due to changes in market conditions or macroeconomic factors.
Limitations of Historical Data: Past data may not accurately represent future conditions. Additionally, the quality and accuracy of the data used in backtesting are critical. Errors or inaccuracies in the data can lead to misleading backtest results.
Backtesting in Practice
There are various ways to perform backtesting, ranging from simple spreadsheet calculations to sophisticated software applications. Numerous commercial and open-source software allow traders and researchers to backtest their strategies with ease.
Enhancing Backtesting Practices
While backtesting offers considerable advantages, it is important to note that rigorous backtesting practices can greatly enhance its efficacy. Here are a few recommendations:
Out-of-Sample Testing: This involves testing your strategy on a set of data that was not used during the development phase. If your strategy performs well during out-of-sample testing, it increases the likelihood that it will perform well on new data.
Cross-Validation: A popular method in machine learning, it involves dividing your data into subsets and running multiple rounds of backtests, each time using a different subset for testing and the remainder for training. This can help ensure your strategy is robust and not just tailored to a specific dataset.
Use of Multiple Metrics: While most backtests focus on net profit or return on investment (ROI), it's important to consider other metrics like Sharpe ratio, maximum drawdown, and trade win rate. These can provide a more holistic view of strategy performance.
Walk-Forward Analysis: This is an iterative form of backtesting that aims to simulate the real-world application of a strategy. It involves optimizing a strategy on a certain period ("in-sample"), then testing it on the following period ("out-of-sample"), and repeating this process through the data.
Sensitivity Analysis: This involves changing the parameters of a strategy to see how sensitive its performance is to those changes. If performance varies widely with small parameter changes, the strategy might be overfit to the data and less likely to perform well in the future.
Consideration of Transaction Costs: Real-world trading involves transaction costs such as broker fees and slippage. It is important to include these in backtests to ensure that profits are not overstated.
Future of Backtesting
Advancements in technology are enabling more comprehensive and accurate backtests. High-performance computing allows for the testing of strategies over large datasets and multiple parameter sets. Artificial intelligence and machine learning are being applied to develop and refine strategies, while blockchain technology can ensure the integrity and accuracy of historical data. Moreover, the emergence of alternative data (data beyond traditional price and volume data, such as social media sentiment, satellite imagery, etc.) allows for the development and testing of novel strategies that were previously unfeasible. As finance continues to evolve, backtesting will undoubtedly remain an essential tool for traders and financial professionals.
Backtesting is an essential component of any successful trading strategy. However, it's only as good as the data, assumptions, and statistical tests that it's built on. It's crucial to understand and respect its limitations and to use it as one tool among many in a financial professional's toolbox. Understanding backtesting can provide a competitive edge and, when used judiciously, can help optimize and validate trading strategies, manage risk, and build confidence in one's trading approach.
Intriguing aspect of backtesting lies in its historical relevance. The concept of backtesting became popular with the advent of modern computers, but its roots can be traced back to centuries-old practices. Ancient merchants and money lenders would often assess their business strategies based on historical trade and loan repayments. While this process was likely far from the systematic, algorithmic backtesting performed today, it involved a similar principle of learning from past performance to refine future strategies. Thus, while technology has radically transformed backtesting, its core concept has been used for centuries, underpinning its fundamental importance in strategy development and validation.
Comments