Counterfactual Reasoning in Causal AI: A Guide for Investors
- Aki Kakko
- Dec 19, 2023
- 3 min read
Updated: Feb 22, 2024
Counterfactual reasoning in Causal AI represents a significant leap in how artificial intelligence understands and interacts with the world. For investors, it's crucial to grasp how this technology can revolutionize various industries and offer substantial investment opportunities. This introduction to Counterfactual Reasoning in Causal AI aims to provide investors with a foundational understanding of the technology's potential and considerations.
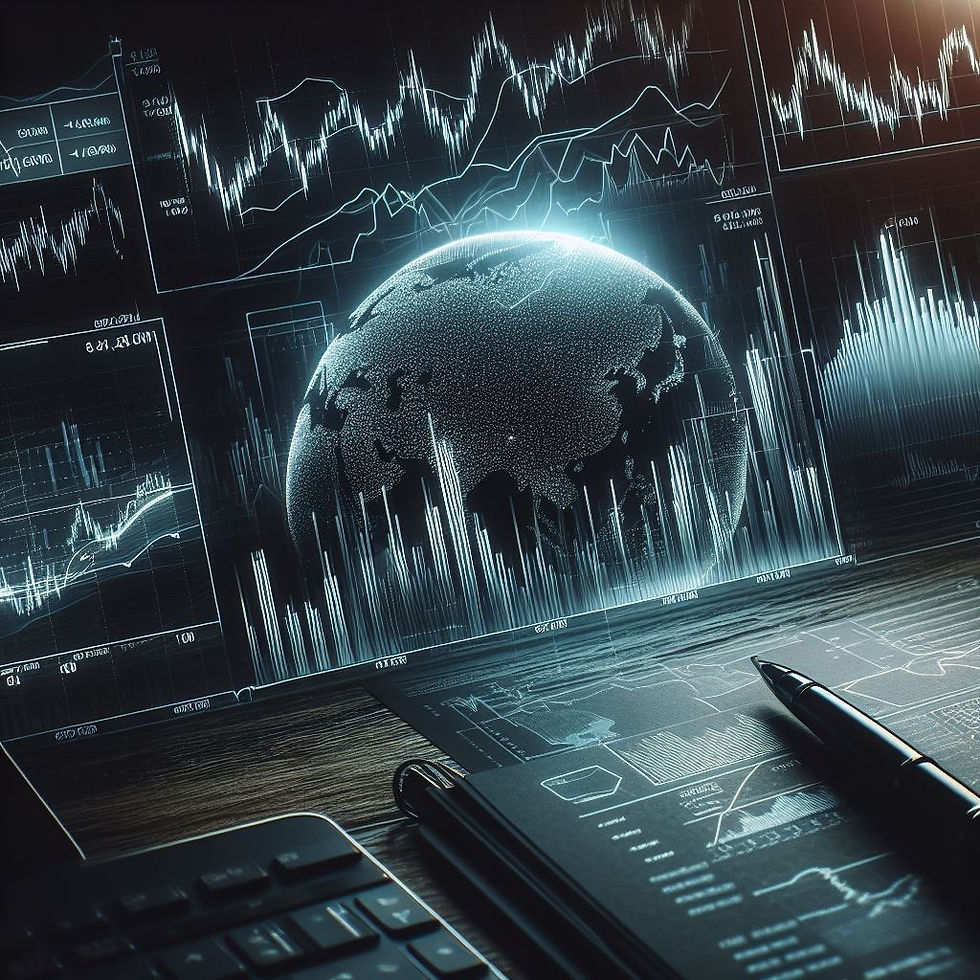
Understanding Counterfactual Reasoning in AI
Counterfactual reasoning involves considering "what if" scenarios. It allows an AI to hypothesize different outcomes based on varying inputs or conditions, going beyond mere correlation to understand causation. Traditional AI models often rely on correlations found in data. However, Causal AI, equipped with counterfactual reasoning, seeks to understand the causal relationships between different variables. Machine learning algorithms are integral to Causal AI, as they help in identifying patterns and relationships in large datasets. However, the addition of counterfactual reasoning allows these algorithms to not just predict outcomes based on historical data but to simulate potential outcomes based on hypothetical scenarios. Incorporating expert knowledge in specific domains (like biology in healthcare or macroeconomics in finance) can significantly enhance the effectiveness of Causal AI models. This integration allows for more accurate and realistic counterfactual analyses.
Applications in Various Industries
Healthcare: In drug development and personalized medicine, Causal AI can predict how different treatments might work on different genetic profiles. For example a biotech firm can use Causal AI to develop a new cancer drug, reducing the time and cost of clinical trials.
Finance: In stock market analysis, it can assess the potential impact of various economic events on stock prices. For example an investment firm can employ Causal AI to model the impact of political events on market volatility, enhancing their risk management strategies.
Retail: It can predict how changes in marketing strategies might affect consumer behavior. For example a retail chain can implement Causal AI to optimize their supply chain, leading to improved stock management and reduced costs.
Manufacturing: Causal AI can foresee the implications of altering manufacturing processes on product quality and efficiency.
Investment Strategies for Causal AI
Diversification Across Sectors: Investing across various sectors that use Causal AI is wise. This diversification can mitigate risks associated with the technology’s infancy and sector-specific challenges.
Long-term Horizon: Causal AI is a groundbreaking yet evolving field. Investors should adopt a long-term perspective, acknowledging that significant advancements and returns may unfold over extended periods.
Collaboration with Academia: Engaging with academic institutions leading in AI research can provide early insights into emerging trends and technologies in Causal AI, offering a competitive edge in investment decisions.
Bias and Fairness: Causal AI must be carefully designed to avoid biases in data and algorithms, which can lead to unfair or unethical outcomes. Investors should be cognizant of the ethical implications of their investments in AI.
Transparency and Accountability: Investing in companies (such as Alphanome) that prioritize transparency in their AI models and algorithms is crucial. Understanding how decisions are made and being able to explain them is vital, especially in sectors like healthcare and finance.
Privacy Concerns: With the increasing importance of data in Causal AI, investing in companies that uphold strict data privacy standards is essential to mitigate risks related to data breaches and privacy violations.
Future Outlook
Future developments in Causal AI are likely to focus on algorithms capable of continuous learning and adaptation, further enhancing their predictive and counterfactual reasoning capabilities. The convergence of Causal AI with other technologies for secure data management or quantum computing for enhanced processing capabilities presents exciting future prospects. Causal AI has the potential to address global challenges, from climate change prediction to managing pandemics. Investments in these areas not only promise financial returns but also contribute to societal well-being.
Risks and Considerations
Complexity and Maturity of Technology: The field is complex and still developing, which can lead to unforeseen challenges.
Data Quality and Availability: The accuracy of Causal AI models depends on the quality and quantity of data.
Regulatory Environment: Changes in data privacy and AI regulations can impact the development and use of Causal AI.
The realm of Counterfactual Reasoning in Causal AI is rich with opportunities for investors. By understanding its capabilities, applications, and implications, investors can make informed decisions that not only yield returns but also propel technological progress and societal benefits. As the field evolves, staying abreast of latest developments and maintaining ethical standards will be key to successful investing in this innovative and impactful technology.
Comments