"P-hacking," or "data dredging," is a critical issue that investors should be aware of when evaluating research, particularly in the realms of finance, economics, and biotech. This practice can lead to misguided investments based on flawed data. Understanding p-hacking and how to identify it can help investors make more informed decisions.
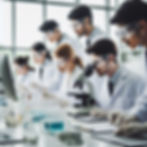
What is P-Hacking?
P-hacking occurs when researchers manipulate their analysis until they find statistically significant outcomes. This process often involves:
Multiple Comparisons: Testing numerous variables and only reporting those that show significant results.
Data Mining: Continuously adjusting data or testing hypotheses until something statistically significant emerges.
Selective Reporting: Omitting certain data points or results that don't support the desired outcome.
Why It's Problematic for Investors
Investors often rely on research to guide their decisions. P-hacked studies can lead to the following issues:
False Positives: Investments based on results that are not genuinely significant or replicable.
Misleading Trends: Believing in trends or correlations that are actually the product of random chance.
Resource Misallocation: Diverting funds to ventures or projects based on unreliable data.
Examples of P-Hacking in Research
Biotech Research: A study claims a new drug significantly reduces disease symptoms, but the result is based on testing numerous dosages and only highlighting the one successful outcome.
Financial Modeling: An analyst reports a model that predicts stock market trends, but the model was derived from numerous trial-and-error methods, adjusting variables until some showed significance.
Behavioral Economics: Research indicating a strong correlation between consumer behavior and economic indicators might be a result of testing numerous indicators and only reporting those that fit the hypothesis.
Economic Growth Research: A study might claim a strong correlation between certain fiscal policies and economic growth. However, if the study tested numerous policy variables and economic indicators, the significant results might be due to chance rather than a true causal relationship.
Consumer Behavior Analytics: Market research suggesting a link between social media trends and consumer purchasing habits could be a result of p-hacking if the researchers tested a vast array of social media metrics before finding a statistically significant one.
How Investors Can Spot P-Hacking
To guard against p-hacking, investors should:
Look for Replication: Trust studies that have been replicated with similar results.
Scrutinize the Methodology: Be wary of studies with a large number of variables but few clear hypotheses.
Seek Transparency: Favor research that openly discusses data collection methods and acknowledges limitations.
The Role of Peer Review and Publication Bias
The effectiveness of peer review in catching p-hacking is mixed. While peer review is supposed to ensure the quality and integrity of research, it often falls short in detecting p-hacked studies, especially if the reviewers are not vigilant about statistical manipulations. Additionally, there's a publication bias towards positive results, which incentivizes p-hacking. Journals are more likely to publish studies with significant findings, which can encourage researchers to manipulate data until they find a significant result.
Advanced Statistical Techniques to Combat P-Hacking
Investors should be aware of advanced statistical techniques that can mitigate the risks of p-hacking:
Pre-registration of Studies: Researchers register their hypothesis, methodology, and analysis plan before conducting research, which limits the scope for data manipulation.
Bonferroni Correction: A statistical method that adjusts significance thresholds when multiple comparisons are made, reducing the likelihood of false positives.
Bayesian Statistics: Unlike traditional methods, Bayesian statistics can incorporate prior knowledge and provide a more nuanced understanding of the likelihood of hypotheses being true.
The Importance of Contextual and Sector Knowledge
Investors should not solely rely on statistical significance. Understanding the context and sector in which the research is conducted is crucial. For instance, in biotech, understanding the biological plausibility of a research finding is as important as the statistical results. In finance, knowledge of economic theory and market conditions can help assess the credibility of research findings.
P-hacking is a significant threat to the integrity of research and can lead to costly mistakes for investors. By being aware of what p-hacking is, understanding how to spot it, and valuing transparency and replication in research, investors can better navigate the complex landscape of investment research and make more informed decisions. Ultimately, blending statistical vigilance with sector-specific knowledge is key to discerning valuable insights from misleading data.