Bayesian Inference in AI: A Guide for Investors
- Aki Kakko
- Sep 12, 2023
- 3 min read
Updated: Mar 16, 2024
Bayesian inference is a method of statistical reasoning that's based on the Bayes theorem. It allows one to update the probability estimate for a hypothesis as more evidence or data becomes available. As an investor, you might wonder, "What does this have to do with AI and why should I care?" Given the rising prominence of AI in financial sectors, it's critical to understand how Bayesian inference shapes AI applications and can guide investment decisions.
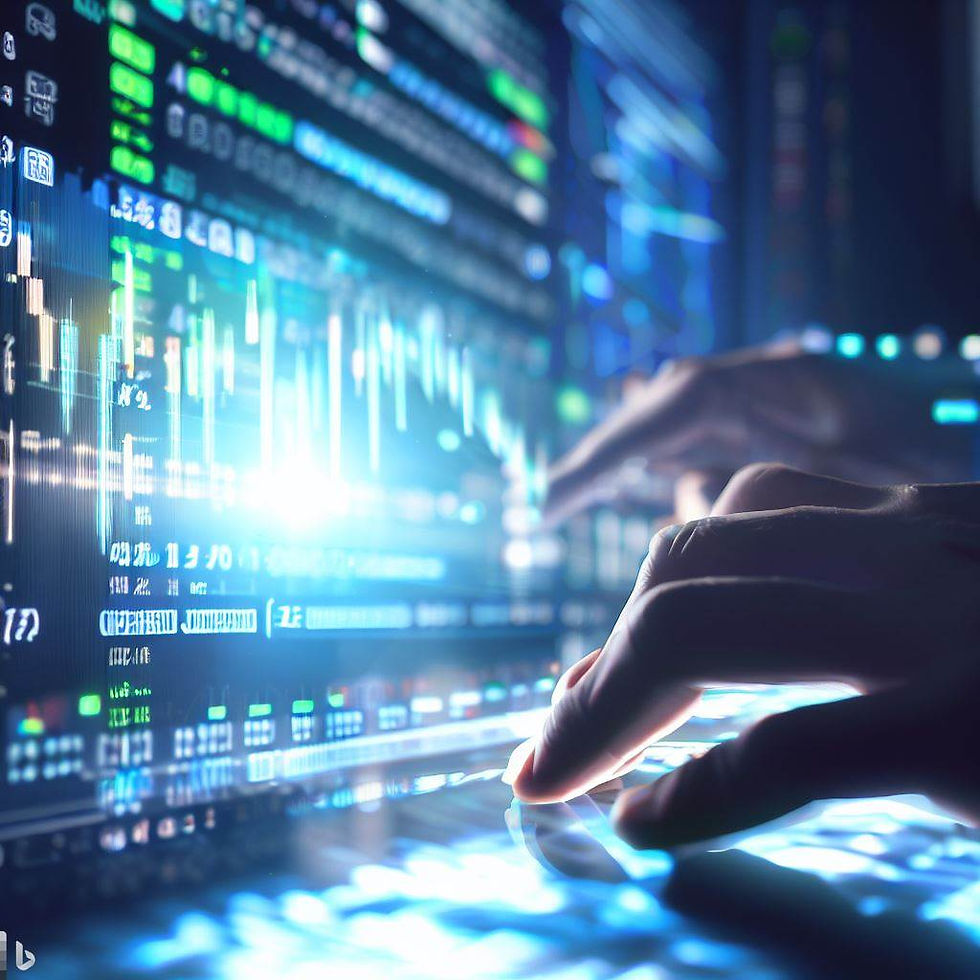
A Simple Explanation of Bayesian Inference
At its heart, Bayesian inference is about updating beliefs based on new evidence. Imagine you're trying to predict the success of a startup. You have an initial belief or estimate about its chances. As you gather more data, like quarterly revenue or user growth, you refine and update your belief about that startup's trajectory.
How AI Uses Bayesian Inference
Predictive Analytics: Many financial institutions use AI-powered predictive analytics to forecast stock prices, currency exchange rates, or other financial metrics. In this context, Bayesian inference can continually refine predictions as more data (like recent stock prices or economic indicators) becomes available. Example: An AI application predicts a particular stock will rise in value. However, a sudden economic downturn affects the market negatively. As the new data pours in, the AI, using Bayesian techniques, recalculates and may then predict a decline for the same stock.
Risk Assessment: Bayesian models are particularly suited for estimating the probability of rare events since they can update their predictions as more data becomes available. Example: A bank might use AI to predict the likelihood of loan defaults. If a region starts showing a higher-than-average default rate, the Bayesian model can adjust its predictions for new loan applicants from that area.
Portfolio Optimization: AI tools can help investors decide how to allocate assets in a portfolio by predicting the future performance of various assets. Bayesian models enhance this by considering prior performance and continually adjusting based on new data. Example: An AI-driven robo-advisor allocates a certain percentage of a portfolio in tech stocks. However, with new data showing potential regulatory challenges for tech companies, the Bayesian model might adjust this allocation, recommending a reduced exposure.
Personalized Banking and Trading: Modern banking often relies on personalizing services for individual customers. AI applications, using Bayesian inference, can predict customer needs or preferences based on their transaction history and adjust as more transactions are made. Example: A trading app might suggest particular stocks to a user. As it learns more about the user's trading patterns and preferences, it refines these suggestions, offering more tailored advice over time.
Startup Investing: One of the most challenging sectors in the investment landscape is startups, given their high risk and potential for high reward. Bayesian inference in AI can be a game-changer here by assessing the probability of a startup's success based on various factors. Example: Imagine an AI tool designed for venture capitalists (VCs). This tool evaluates startups based on a myriad of data points: the industry they're in, the background of the founders, the current market demand, etc. Initially, a startup might have a certain probability of success based on these factors. However, as the startup progresses and new data becomes available—maybe it secures a significant partnership, or perhaps a competitor emerges—the AI tool, leveraging Bayesian inference, recalibrates its predictions. This allows VCs to make more informed decisions, whether it's deciding to invest, determining the valuation, or choosing to exit.
Why Investors Should Care
Data-Driven Decisions: Bayesian models make AI tools more adaptive. As an investor, aligning with platforms or services that employ these methods means you're leveraging some of the most adaptive tools available.
Managing Uncertainty: The financial world is fraught with uncertainties. Bayesian models, by their very nature, are designed to handle uncertainty, recalculating risks and rewards as new data emerges.
Future-Proofing: As the financial world becomes more complex and interlinked, the tools that can best handle vast amounts of changing data will be invaluable. Bayesian models are inherently suited for this task.
Efficiency: Bayesian-based AI applications often require fewer data to make accurate predictions. For investors, this could mean faster insights and more agile decision-making.
Bayesian inference is more than just a statistical method; it's a framework for updating beliefs in the face of new information. As AI continues to revolutionize the investment landscape, understanding and leveraging the power of Bayesian techniques can provide investors with a significant advantage. Whether you're making personal investment decisions or steering the course of a major financial institution, the adaptability and precision of Bayesian-informed AI tools can be invaluable assets.
Comments