Embeddings Based Retrieval for Investors
- Aki Kakko
- Oct 9, 2023
- 3 min read
Updated: Feb 13, 2024
In today's digital age, vast amounts of data are generated every second. For investors and businesses alike, making sense of this data is critical. Enter the world of embeddings based retrieval — a powerful method to extract meaningful insights from data, especially text. In this article, we'll dive into what embeddings based retrieval is, why it's relevant for investors, and provide concrete examples of its application.
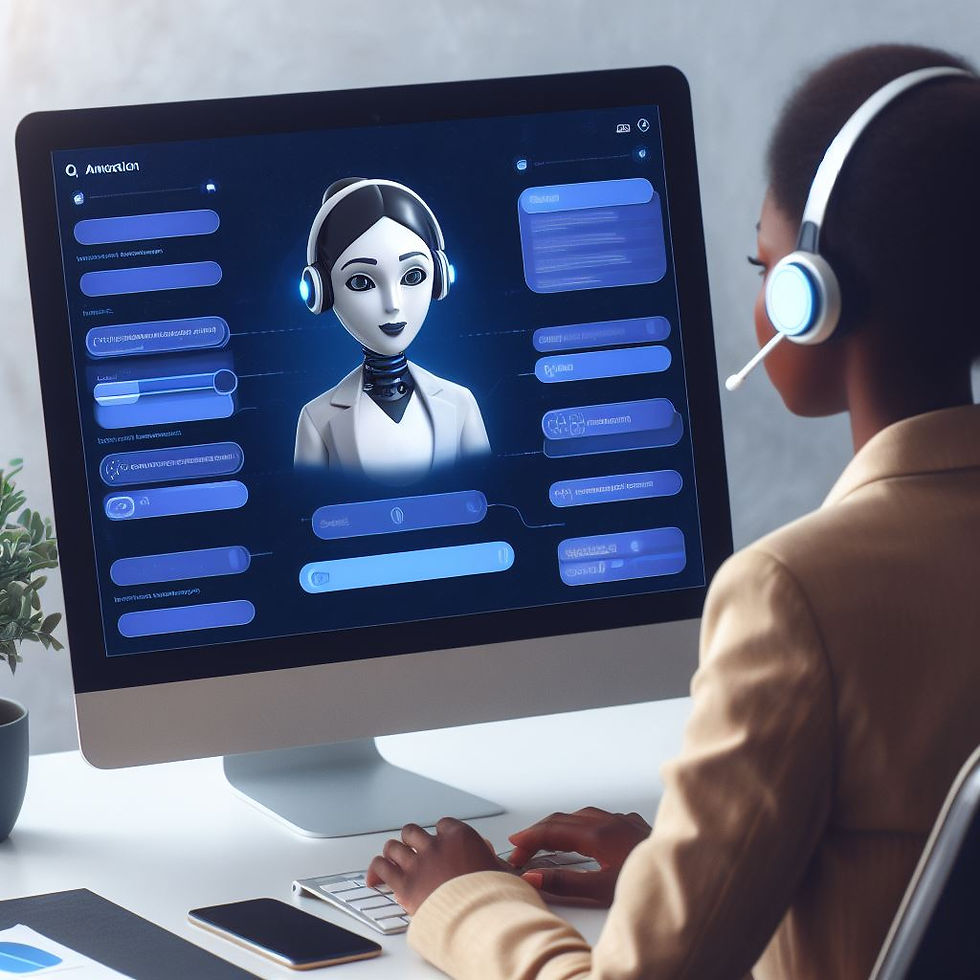
What is Embeddings Based Retrieval?
At its core, embeddings based retrieval is about representing data in a way that similar items are close together in a high-dimensional space. In simpler terms, embeddings are a type of data representation where items (like words, products, or stocks) are mapped to vectors of real numbers. The proximity of these vectors signifies their similarity. Embeddings can be derived from various techniques, such as Word2Vec, GloVe, BERT, and more. Once items are represented as vectors, they can be quickly compared and retrieved based on their similarity.
The Science Behind Embeddings
At the foundation of embeddings is the idea that words or items occurring in similar contexts share semantic meaning. Models like Word2Vec achieve this by training on vast amounts of text. As these models encounter words together in sentences, they adjust the vectors representing those words to bring them closer together in the embedding space.
Transfer Learning
Recent advances in natural language processing, particularly models like BERT and GPT, allow for transfer learning. This means pre-trained models can be fine-tuned on specific financial datasets to generate even more relevant embeddings for investors.
Why is it Relevant for Investors?
Information Overload: Investors are swamped with news articles, financial reports, and other textual data. Embeddings can help summarize, categorize, and draw connections between seemingly unrelated pieces of information.
Quantitative Analysis: Many investors rely on quantitative strategies. Embeddings can transform unstructured data into structured forms that can be fed into machine learning models or other quantitative tools.
Risk Management: Understanding relationships between assets is crucial for risk management. Embeddings can reveal hidden correlations between different stocks or sectors.
Examples of Embeddings Based Retrieval in Investment:
News Sentiment Analysis: Consider an investor tracking news about a particular stock or sector. With embeddings, news articles can be converted into vectors. By comparing these vectors with pre-defined sentiment vectors (e.g., "positive", "negative"), the investor can gauge the overall sentiment of news articles about the stock. Example: An investor wants to gauge sentiment around Company A after their earnings report. By using embeddings, they can quickly sift through hundreds of news articles, highlighting ones that lean negative or positive, helping them make a more informed decision about their investment.
Financial Document Retrieval: Investors often need to refer to specific sections of lengthy financial reports. Embeddings can help retrieve the most relevant sections based on a query. Example: An investor is looking for details about Company B's overseas revenues. Instead of manually scanning the entire annual report, they can use an embeddings-based search to quickly retrieve the section discussing overseas revenue.
Portfolio Diversification: By representing stocks as vectors based on various factors (historical performance, news sentiment, etc.), investors can identify stocks that are similar or dissimilar. This can be useful in creating a diversified portfolio. Example: An investor wants to ensure their tech portfolio isn't overly concentrated in one sub-sector. Using embeddings, they could visualize the similarity between different tech stocks and adjust their holdings accordingly.
Prediction Models: Embeddings can be fed into machine learning models to predict stock prices, future earnings, or any other financial metric. Example: An investor has access to thousands of analyst reports. Using embeddings, they can convert these reports into structured data, which can then be used to predict the next quarter's earnings for a particular stock.
Challenges and Considerations:
Quality of Data: The effectiveness of embeddings heavily relies on the quality of data. Noise or irrelevant data can lead to misleading embeddings. It’s crucial to curate and preprocess data adequately.
Interpretability: While embeddings can capture complex relationships, they aren't always straightforward to interpret. Advanced visualization techniques, like t-SNE, can help, but understanding why two vectors are close in the embedding space can sometimes be more art than science.
Computational Costs: Generating and working with embeddings, especially in real-time scenarios, can be computationally expensive. Investors need to balance the benefits against the computational and time costs.
Embeddings based retrieval is a powerful tool in the hands of modern investors. By transforming unstructured data into a structured form, it offers a pathway to gain deeper insights, make more informed decisions, and optimize investment strategies. As the world of finance becomes increasingly data-driven, understanding and leveraging technologies like embeddings will be critical for sustained success.
Comments