Knowledge Distillation: A Primer for Investors
- Aki Kakko
- Aug 17, 2023
- 4 min read
Updated: Mar 16, 2024
The rapid growth of machine learning, particularly deep learning, has introduced a range of novel techniques aimed at improving the performance, efficiency, and generalization of models. One such technique that has gained traction is knowledge distillation. This article will delve into what knowledge distillation is, its significance, and how it can be advantageous for businesses and investors.
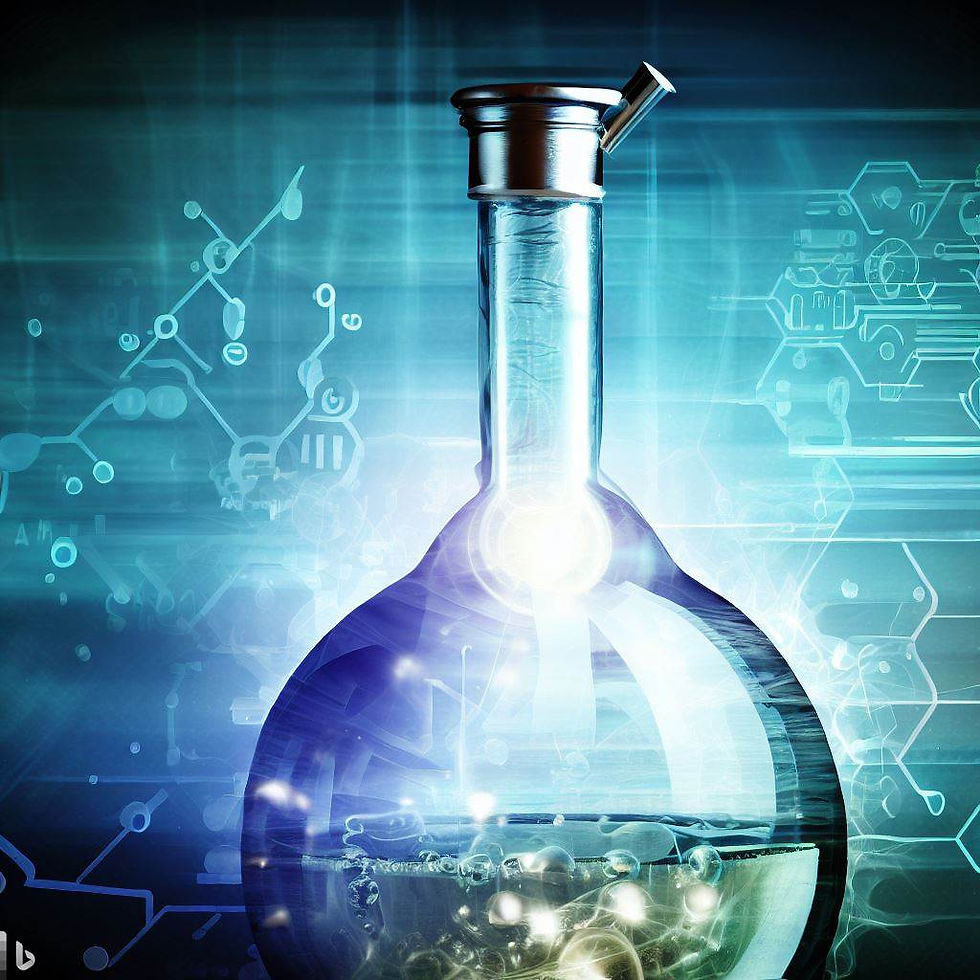
What is Knowledge Distillation?
Knowledge distillation (KD) is a process in which a smaller model (often called the "student" model) is trained to mimic the behavior of a larger, often more complex model (known as the "teacher" model). The aim is to transfer the "knowledge" from the teacher model to the student model. In a conventional training scenario, models learn directly from data. However, with KD, the student model learns from the outputs of the teacher model. The intuition is that the teacher, being a larger model, has captured intricate patterns in the data, which can be distilled into the student model without it having to be of the same size or complexity.
Why is Knowledge Distillation Important?
Efficiency: Deep learning models, particularly those with millions or billions of parameters, can be computationally expensive and slow, especially on devices with limited resources. KD allows us to have smaller models that are faster and consume less memory, making them ideal for deployment on edge devices, mobile phones, and embedded systems.
Cost-effective: Training large models demands considerable computational resources and can be costly. By distilling knowledge into smaller models, businesses can reduce operational costs.
Model Generalization: A distilled model may generalize better in some scenarios by avoiding overfitting, which is a common problem with very large models.
Practical Examples of Knowledge Distillation
Mobile Applications: For apps that use image recognition, deploying a large neural network directly on the phone might be inefficient. Using KD, companies can create a smaller version of a well-trained image recognition model that runs smoothly on mobile devices without compromising much on accuracy.
IoT Devices: In the realm of the Internet of Things, devices often need to make real-time decisions based on data they capture. A smaller distilled model can make these decisions quickly without the need for constant communication with a central server.
Medical Devices: Portable diagnostic devices, such as those used for detecting diabetic retinopathy from retinal images, can benefit from lightweight models that offer quick diagnostic results.
Considerations for Investors
Intellectual Property: As KD becomes more prevalent, there will be an increase in IP creation around efficient distillation techniques. Investors should be aware of the patent landscape and the potential for competitive differentiation through unique KD approaches.
Shift in Model Deployment Strategies: Companies might transition from deploying large models in centralized servers to deploying smaller models at the edge. This can impact the infrastructure and cost dynamics of businesses.
Training Data Sensitivity: KD can sometimes obfuscate the training data used for the teacher model. This can be especially pertinent in sectors where data privacy and security are paramount.
Potential for New Business Models: Companies specializing in training large models could potentially offer their models as teachers for others to distill, leading to a new marketplace dynamic.
Knowledge distillation presents an opportunity to harness the power of large, complex models in smaller, more efficient packages. For investors, understanding this technique and its implications can offer insights into future trends in technology deployment, cost-saving opportunities, and potential growth areas in the AI and machine learning sectors. As with all technological innovations, the key lies in discerning its practical applications and the value it brings to businesses and end-users.
Knowledge Distillation in Investing
In the investing world, data-driven decisions are paramount. Advanced machine learning models are frequently used to extract patterns, predict market movements, and identify investment opportunities. Knowledge distillation can play a transformative role in this sector. Here's how:
Real-time Trading Systems: Large and complex trading models, while potentially more accurate, can be slow to make predictions or decisions due to their size. In the world of high-frequency trading, a fraction of a second can make a significant difference. A distilled model, with its faster inference time, can be used to execute trades at a quicker rate, ensuring that opportunities are not missed.
Portable Analysis Tools: Financial analysts and portfolio managers often rely on data analysis tools that use machine learning. However, not all tools can be accessed in real-time, especially on mobile devices, due to the sheer computational requirements of complex models. Distilled models can be embedded into mobile applications or lightweight desktop software, giving professionals the ability to make data-driven decisions on-the-go without a compromise on accuracy.
Reduced Infrastructure Costs: Maintaining the infrastructure for running large-scale models can be costly, especially with the need for high-performance servers and extensive memory. By utilizing distilled models that require less computational power, investment firms can save on infrastructure costs, both in terms of hardware and energy consumption.
Data Privacy and Security: Investment strategies, trading patterns, and financial data are proprietary and sensitive. Transferring data to centralized servers for analysis by large models can pose security risks. By deploying distilled models at the edge, closer to the data source, data transmission can be minimized, reducing the risk of interception or breaches.
Ensemble Learning: Investment decisions often benefit from multiple models or perspectives. An ensemble of models can provide a more holistic view but can be computationally heavy. Knowledge distillation can be used to distill the ensemble's knowledge into a single, lightweight model. This distilled model can offer the combined insights of the ensemble but with the efficiency of a singular model.
Personalized Investment Strategies: Retail investors often don’t have access to sophisticated investment models due to computational limitations. Investment platforms can use knowledge distillation to offer personalized investment strategies. A large model trained on global financial data can be distilled to create personalized models for individual investors based on their portfolio and risk preferences.
In the realm of investing, where timely and accurate decisions are of the essence, the efficiency of knowledge distillation offers a compelling advantage. Investment firms and fintech startups can leverage this technique not only to enhance their decision-making processes but also to democratize access to advanced investment tools for a broader audience. As the investing world increasingly embraces AI-driven tools, knowledge distillation stands out as a pivotal technique for the next generation of financial innovation.
Commentaires