Understanding Spillover Effects in Causal Inference for Investors
- Aki Kakko
- Nov 30, 2023
- 3 min read
Updated: Mar 16, 2024
Spillover Effect Analysis in Causal Inference is a crucial concept for investors, especially those involved in portfolio management and market analysis. This concept is integral to understanding how an intervention or event in one part of the economy can indirectly affect other parts, impacting investment decisions.
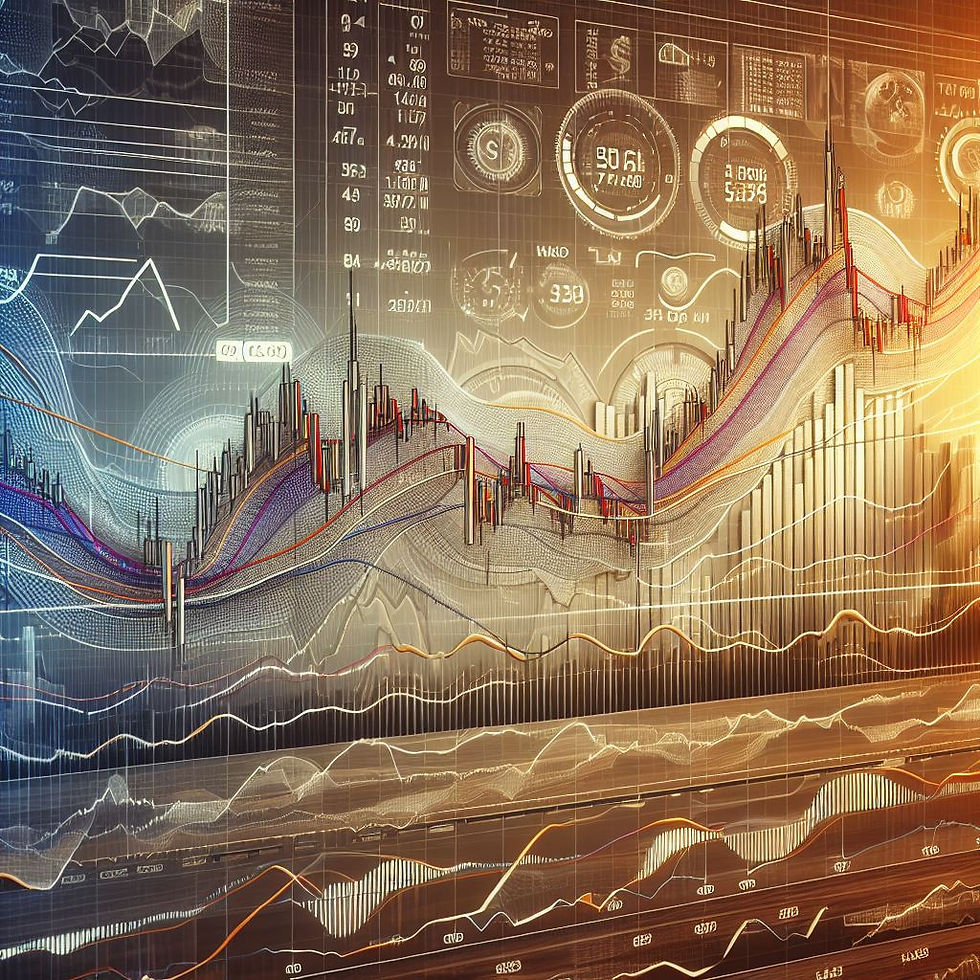
Understanding Spillover Effects
A spillover effect occurs when an event or policy in one sector or market influences another unrelated sector or market. This can be due to various factors like investor behavior, economic linkages, or global trends. For instance, a regulatory change in the technology sector might impact consumer behavior, thereby affecting the retail sector.
Importance for Investors
Risk Management: Understanding spillover effects helps in identifying hidden risks in a portfolio. An investor might think they are diversified, but spillover effects can create unexpected correlations between seemingly unrelated assets. By anticipating potential spillovers, investors can proactively manage risks, including using financial derivatives or hedging strategies to protect their portfolios.
Strategic Allocation: By anticipating spillover effects, investors can position their portfolios to benefit from these indirect influences. This might involve investing in sectors that stand to benefit from certain policies or trends in other sectors. Investors can use spillover effect analysis to adjust their asset allocation dynamically in response to changing market conditions.
Portfolio Diversification: Understanding spillover effects can lead to more effective diversification strategies, helping investors mitigate risks that are not apparent on the surface.
Examples of Spillover Effects
Tech Regulation and Retail Market: Increased regulation in the tech industry, such as privacy laws, can impact digital advertising. This, in turn, affects the retail sector's marketing strategies and consumer reach, ultimately impacting retail stocks.
Oil Prices and Automobile Industry: A rise in oil prices can lead to increased costs for transportation companies, which might reduce their profitability. This could indirectly affect the automobile industry as consumers may prefer fuel-efficient or electric vehicles, boosting stocks in these sectors.
Interest Rate Changes and Real Estate: Central bank policies on interest rates can have a significant spillover effect. An increase in interest rates can make mortgages more expensive, potentially slowing down the real estate market. This can have further effects on construction, home goods retailers, and financial services.
The 2008 Financial Crisis: The collapse of the housing market in the United States had a massive spillover effect globally, impacting financial markets, economies, and sectors far beyond real estate.
COVID-19 Pandemic: The pandemic led to significant spillover effects, such as the surge in e-commerce benefiting the tech sector and impacting retail, logistics, and real estate markets.
Analyzing Spillover Effects
Econometric Models: These models can quantify the extent of spillover effects between different sectors or markets. They use historical data to identify patterns and correlations that might not be immediately apparent.
Sentiment Analysis: Understanding investor sentiment through news, social media, and financial reports can provide insights into potential spillover effects. For example, negative sentiment in one industry can lead to a lack of confidence in related sectors.
Scenario Analysis: This involves assessing how different events (like a change in government policy) could create spillover effects across various sectors. It helps in preparing for potential market shifts.
Advanced Techniques in Spillover Effect Analysis
To deepen their understanding of spillover effects, investors can employ several advanced techniques:
Network Analysis: This involves mapping the relationships between different sectors or assets to understand how changes in one area might affect others. For example, network analysis can reveal how tech companies are interconnected with various sectors like finance, retail, and manufacturing.
Machine Learning Models: These models can process vast amounts of data to identify complex, non-linear spillover effects that traditional econometric models might miss. They can predict potential spillovers by analyzing patterns in market data, news, and economic indicators.
Cross-Market Analysis: Investors can analyze data from different markets (like stocks, bonds, commodities) to understand how movements in one market can affect others. This is particularly important in a globalized economy where markets are increasingly interconnected.
Challenges in Spillover Effect Analysis
Despite its importance, analyzing spillover effects comes with challenges:
Data Overload: The sheer volume of data can be overwhelming, and identifying relevant data for spillover analysis is critical.
Prediction Uncertainty: Predicting spillover effects is inherently uncertain, especially in the face of unprecedented events like geopolitical crises or pandemics.
Dynamic Markets: Markets are constantly evolving, and past spillover patterns might not always predict future effects accurately.
Spillover Effect Analysis in Causal Inference offers a comprehensive approach for investors to understand and anticipate the indirect impacts of various events and policies on different sectors and markets. By incorporating this analysis into their investment strategy, investors can better manage risks and identify potential opportunities in a dynamic market environment. Understanding and anticipating these effects requires a combination of quantitative analysis, market insight, and a keen eye on global trends and policies. Investors who master this aspect of market analysis can significantly improve their decision-making process, leading to more robust and resilient investment strategies.
Comments