Are Our "Agentic" AIs Actually Antetic? A Deep Dive into the Illusion of Individual Agency
- Aki Kakko
- Mar 12
- 4 min read
Updated: Apr 5
The current wave of excitement surrounding "Agentic AI" paints a picture of independent, autonomous AI entities proactively pursuing goals and making decisions. However, a closer examination of these systems reveals a potential disconnect between the perception of agency and the underlying architecture. Are these so-called agentic AIs truly autonomous individuals, or are they, in essence, complex ant colonies disguised as singletons? This article delves into the intricacies of agency, dissects the architecture of current "agentic" systems, and argues that many, in fact, operate closer to an Antetic model than we realize.
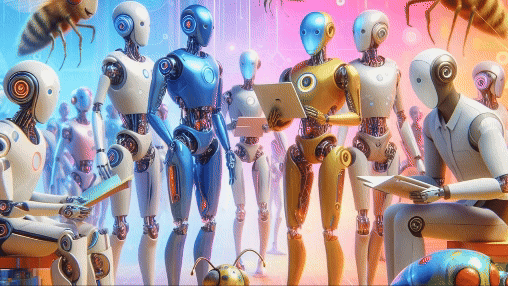
The Allure of Agency: Why We Want Independent AI
The concept of an "agent" in AI implies several key characteristics:
Autonomy: The ability to operate without constant human intervention.
Proactiveness: The capacity to initiate actions to achieve goals.
Goal-Directedness: A clear understanding of objectives and a drive to achieve them.
Adaptability: The ability to learn from experience and adjust behavior accordingly.
Rationality: Making decisions based on logic and available information.
The promise of agentic AI is immense: autonomous vehicles navigating complex traffic, personal assistants proactively managing our schedules, AI systems autonomously driving scientific discovery. These visions hinge on the belief that we can create AI entities that act independently and intelligently in pursuit of defined goals.
The Antetic Undercurrent: How "Agentic" AIs Resemble Ant Colonies
The key argument is that many current "agentic" AIs operate as a collection of relatively simple components working in concert, rather than as a single, truly autonomous entity with integrated reasoning and decision-making capabilities. Here's how:
Modular Architecture and Task Decomposition: "Agentic" AIs typically break down complex tasks into smaller, simpler sub-tasks. Each sub-task is then assigned to a specialized "tool" or module, akin to assigning specific foraging duties to different groups of ants within a colony. This division of labor is a hallmark of Antetic systems.
Chain-of-Thought (CoT) and Reflexion as Stigmergy: The "Chain-of-Thought" prompting technique used in LLMs, where the model explicitly outlines its reasoning steps, can be seen as a form of stigmergy. The LLM's reasoning acts as the environment, guiding its future "thoughts" and actions. Similarly, the "Reflexion" technique, where the agent reflects on past experiences and generates new strategies, is akin to the colony learning from its collective experiences and adapting its behavior. One action creates an environment that is then used by the next action.
LLMs as Pheromone Disseminators: LLMs, while powerful, act as the primary communication mechanism between different components of the "agentic" AI. The text they generate serves as a "pheromone trail," conveying information and influencing the actions of subsequent modules. LLMs are the chemical signal driving actions of the tools. The specialized tools respond to this "pheromone trail," similar to how ants respond to pheromones to find food or navigate back to the nest.
Lack of Integrated Self-Awareness: Current "agentic" AIs lack a unified, self-aware consciousness. Instead, they rely on a series of independent processes that are loosely coordinated by a central controller or framework. This is analogous to the lack of a central authority in an ant colony. Each "ant" (module) performs its assigned task without a comprehensive understanding of the overall goal or the consequences of its actions.
External Guidance and Task Priming: While these AIs can operate autonomously for a certain period, they typically require significant upfront priming from humans in the form of task definition, goal specification, and sometimes even examples of desired behavior. This initial "training" can be viewed as setting the initial conditions for the "ant colony" to operate effectively. This initial starting point is like a human defining where food should be scavenged from instead of the AI learning that.
The Agency Consideration: An Illusion of Control?
The central question is whether this architectural approach truly constitutes agency. While the "agentic" AIs can perform complex tasks autonomously, their actions are largely determined by the pre-defined modules, communication mechanisms, and task decomposition strategies. This suggests that what we perceive as agency might be an illusion created by the emergent behavior of a complex, but ultimately pre-programmed, system. The output is agency, but the input is not. We might be anthropomorphizing the systems and applying our understanding of human agency inappropriately. Just because an AI can make a decision does not mean it is behaving as an independent agent, making conscious choices.
The Implications of Antetic AI masquerading as Agentic AI:
Understanding the true nature of these "agentic" AIs has several important implications:
Risk Mitigation: Recognizing that these systems are closer to Antetic than Agentic is crucial for risk mitigation. Focusing on robust communication protocols, error handling, and ensuring that the collective behavior aligns with ethical guidelines becomes paramount. If we are essentially creating a self-organized system, we need to understand how to guide its emergence in a safe and beneficial direction.
Explainability and Interpretability: Understanding the complex interactions within these systems becomes more challenging. Instead of focusing on explaining the decision-making process of a single "agent," we need to analyze the emergent behavior of the entire system. This requires developing new tools and techniques for understanding and interpreting complex, decentralized systems.
True Agency as the Next Frontier: Acknowledging the limitations of current "agentic" AIs paves the way for future research aimed at creating genuinely autonomous AI entities with integrated reasoning, self-awareness, and the capacity for independent thought and innovation.
Reframing Our Expectations and Research Focus
Current "agentic" AIs are impressive technological achievements, but a closer examination reveals that many operate more like complex Antetic systems than truly autonomous agents. By recognizing this underlying architecture, we can better understand their capabilities, limitations, and potential risks. This understanding is essential for navigating the ethical considerations of AI development and for charting a course towards creating genuinely agentic AI systems that embody the full potential of autonomous thought and action. The future may not be about creating isolated digital geniuses, but about building intelligent ecosystems that leverage the power of collective intelligence, mirroring the remarkable success of ant colonies in the natural world. Ultimately, the conversation must shift from simply labeling systems "agentic" to deeply analyzing their underlying architectures and carefully considering the degree to which they truly embody the principles of autonomy, proactiveness, and rationality that define genuine agency. This nuanced perspective will be critical for shaping the future of AI and ensuring that its development aligns with human values and societal goals.
Comentarios