The AntGI Hypothesis: Deciphering Evolutionary Intelligence for a Transformative Path to AGI
- Aki Kakko
- Mar 11
- 6 min read
Updated: Apr 3
The pursuit of Artificial General Intelligence, a machine intelligence that matches or surpasses human cognitive abilities across a spectrum of tasks, has become a central ambition in modern technology. While current AI development heavily emphasizes replicating the structural and functional complexity of the human brain, the AntGI hypothesis proposes a paradigm shift: achieving a deeper understanding of the simpler, yet remarkably effective, intelligence exhibited by ant colonies offers a more fertile and potentially faster route to AGI. The central claim of AntGI is that the key to unlocking the secrets of general intelligence isn’t solely through mimicking the endpoint of evolution (the human brain), but by dissecting the evolutionary journey of intelligence itself. By focusing initially on "Ant Level Intelligence" - not just emulating ant behavior, but comprehensively understanding the mechanisms that allow ant colonies to solve complex problems - AntGI aims to uncover fundamental algorithms and evolutionary principles that underpin all forms of learning, including human cognition. This approach, it argues, provides a solid foundation for developing truly adaptable, robust, and efficient AI systems.
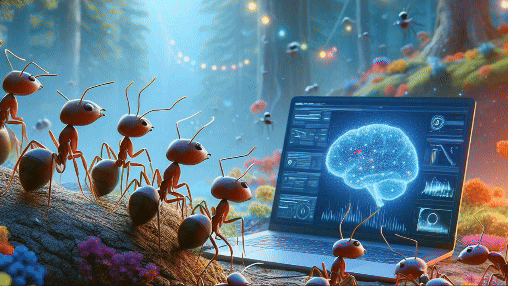
Beyond Individual Ants: The Power of Emergent Colony Intelligence
Ant colonies represent a fascinating example of emergent intelligence. Individual ants are relatively simple organisms, yet their collective behavior results in sophisticated problem-solving abilities that far exceed the capabilities of a single ant. This collective intelligence manifests in various forms:
Dynamic Foraging and Resource Optimization: Ant colonies can efficiently explore vast and changing environments to locate and harvest scarce resources. They employ sophisticated strategies like trail laying (using pheromones to mark paths) and recruitment mechanisms to optimize foraging routes and adapt to fluctuations in resource availability. The colony’s foraging strategy constantly evolves based on feedback from individual ants, creating a self-optimizing system.
Decentralized Decision-Making and Task Allocation: The colony functions without a central command structure. Decisions about tasks such as nest construction, defense, and food allocation are made collectively through distributed communication and interaction. Individual ants assess local conditions and respond accordingly, contributing to a global solution that optimizes colony performance. This decentralized approach makes the colony highly resilient to disruptions.
Complex Nest Construction and Environmental Engineering: Certain ant species build elaborate nests with intricate architectures, demonstrating a remarkable capacity for engineering and construction. These nests often feature specialized chambers for different purposes, advanced ventilation systems, and even temperature regulation mechanisms. The colony's ability to adapt nest design to local environmental conditions highlights their advanced problem-solving skills.
Social Immunity and Disease Management: Ant colonies have evolved sophisticated mechanisms to combat disease. They exhibit behaviors such as grooming, waste removal, and social distancing to prevent the spread of pathogens. Some species even produce antibiotics to protect themselves against infection. These behaviors demonstrate a form of collective immunity that safeguards the colony's health and survival.
Learning and Adaptation to Novel Environments: Ant colonies demonstrate the capacity to learn from experience and adapt to new environments. They can develop new foraging strategies, overcome obstacles, and even learn to exploit novel food sources. This adaptability highlights the colony's inherent capacity for learning and innovation.
Sophisticated Communication Through Chemical Signaling: Ants utilize an intricate system of pheromone communication to coordinate their actions, share information about food sources, warn of danger, and regulate colony behavior. This system allows for complex information processing and transmission within the colony, enabling collective decision-making and efficient task allocation. Different pheromones convey specific messages, creating a sophisticated language that supports the colony's social organization.
The AntGI hypothesis contends that these complex behaviors originate from relatively simple learning rules implemented by individual ants. The crucial insight is that when these rules are combined with communication, interaction, and the selective pressures of evolution, a powerful and adaptive collective intelligence emerges. By isolating, understanding, and replicating these fundamental principles, AntGI proposes a more biologically grounded approach to achieving AGI.
The AntGI Methodology: A Convergence of Disciplines
The AntGI hypothesis necessitates a multi-faceted research strategy that integrates diverse disciplines:
High-Fidelity Agent-Based Modeling (ABM): Developing advanced ABM simulations that accurately model ant behavior, pheromone diffusion, environmental dynamics, and inter-ant interactions. These simulations should incorporate realistic representations of ant sensory perception, locomotion, and social interactions. The goal is to create a virtual "ant farm" where researchers can experiment with different learning rules, communication strategies, and environmental conditions in a controlled and scalable manner. The fidelity of the simulation will be crucial in ensuring that the results accurately reflect real-world ant behavior.
Bio-Inspired Algorithm Development and Machine Learning: Designing novel machine learning algorithms that mimic the mechanisms observed in ant colonies, such as pheromone-based communication, decentralized decision-making, stigmergy (indirect coordination through environmental modification), and adaptive task allocation. This includes going beyond traditional Ant Colony Optimization (ACO) and exploring new approaches inspired by the latest findings in ant biology. The aim is to generalize these ant-inspired algorithms for broader AI applications, moving beyond specific optimization problems to more general learning and reasoning tasks.
Robotic Emulation and Hardware Implementation: Creating physical robots that replicate ant locomotion, sensory perception, and communication. These robots should be capable of interacting with their environment, communicating with each other, and performing tasks collaboratively. This allows for real-world testing and validation of the developed algorithms in complex, dynamic environments. Swarm robotics offers a promising platform for implementing and testing ant-inspired algorithms.
Advanced Neuroscience and Behavioral Ecology Studies: Conducting in-depth studies of ant brain structure and function using advanced neuroimaging techniques and behavioral observation. This involves analyzing neural activity during different tasks, identifying the brain regions involved in learning and decision-making, and studying the communication signals used by ants. Detailed behavioral studies in natural settings are crucial for understanding how ants adapt to their environment and interact with each other in real-world conditions.
Mathematical Modeling and Information Theory: Developing mathematical models to describe the collective behavior of ant colonies and analyze the underlying principles of emergent intelligence. This includes using information theory to quantify the amount of information exchanged between ants, analyzing the efficiency of different communication strategies, and developing models of collective decision-making. These models provide a theoretical framework for understanding how simple individual rules can lead to complex, colony-level behaviors.
Evolutionary Computation and Algorithm Optimization: Applying evolutionary algorithms to optimize the parameters of ant-inspired AI systems, simulating the process of natural selection to improve their performance and robustness. This involves creating a population of AI agents with different parameter settings, evaluating their performance on a set of tasks, and selecting the best-performing agents to reproduce and evolve. This process can be repeated over many generations to create highly optimized AI systems that are well-adapted to their environment.
The integration of these diverse methodologies aims to provide a holistic and comprehensive understanding of ant intelligence, bridging the gap between biology, engineering, and computer science.
Evolutionary Foundations for a Sustainable AGI
The core rationale behind AntGI is that the evolutionary origins of intelligence provide critical insights for developing more robust, adaptable, and sustainable AI systems. By understanding how learning algorithms have evolved over millions of years, AntGI aims to:
Enhance Robustness and Resilience: Develop AI systems that can learn from limited data, adapt to noisy or incomplete information, and handle unexpected changes in their environment, mimicking the resilience of ant colonies.
Improve Energy Efficiency: Nature has optimized learning systems for energy efficiency. Studying these systems could lead to more energy-efficient AI algorithms, crucial for sustainable AI development and deployment.
Unlock New Learning Paradigms: Exploring ant intelligence could reveal novel learning paradigms beyond traditional supervised and unsupervised learning, potentially leading to breakthroughs in AI research. For instance, stigmergic learning, where agents indirectly coordinate through modifications to the environment, could inspire new AI architectures.
Gain a Deeper Understanding of Human Cognition: Unraveling the fundamental building blocks of intelligence refined over millennia provides valuable insights into the evolutionary origins of human cognition and potentially illuminate the path to replicating higher-level cognitive functions in machines.
Accelerate the Path to AGI Through Foundational Principles: By leveraging the proven principles of learning, optimization, and adaptation in nature, AntGI offers a more grounded approach to achieving AGI, potentially bypassing some of the challenges currently hindering its development.
Develop More Explainable AI: Understanding the underlying mechanisms of ant-inspired AI could lead to more transparent and explainable AI systems, addressing a major concern in current AI research. By understanding how individual agents contribute to the collective behavior, we can gain insights into the decision-making processes of the system as a whole.
AntGI: Beyond Biomimicry, Towards Evolutionary AI
The AntGI hypothesis is not merely about creating AI systems that superficially mimic ant behavior. It's about extracting the fundamental principles and mechanisms that drive ant intelligence and applying them to the development of more general-purpose AI systems. By focusing on achieving Ant Level Intelligence as a crucial stepping stone, AntGI offers a potentially more biologically plausible and evolutionarily informed approach to achieving AGI, a transformative approach that has the potential to reshape our understanding of intelligence and unlock new possibilities for the future of AI. By learning from the wisdom of the colony, we might just be able to build a truly intelligent machine.
Comments