Gaussian Process Regression: A Powerful Machine Learning Tool for Investment Forecasting
- Aki Kakko
- Apr 21, 2024
- 4 min read
Investors are constantly seeking reliable methods to analyze historical data and make informed predictions about future market trends. One machine learning technique that has gained significant traction in recent years is Gaussian Process Regression (GPR). This powerful tool offers a flexible and robust approach to forecasting, making it an invaluable asset for investors.
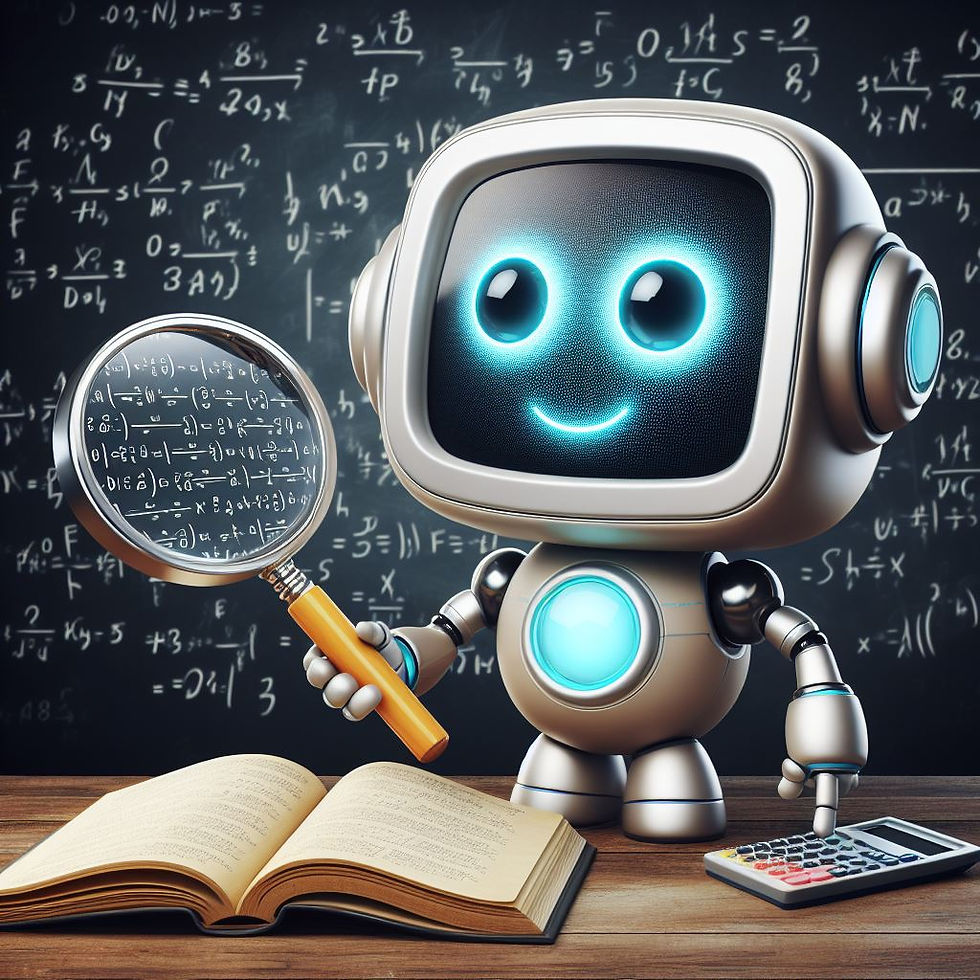
What is Gaussian Process Regression?
Gaussian Process Regression (GPR) is a non-parametric, probabilistic modeling technique used for regression analysis. Unlike traditional regression methods that assume a specific functional form for the data, GPR treats the underlying function as a Gaussian process, which is a collection of random variables with a joint Gaussian distribution. The key advantage of GPR lies in its ability to capture complex, non-linear relationships between input variables and the target variable without relying on predefined models. This flexibility makes GPR particularly well-suited for financial data, where market dynamics can often exhibit intricate patterns and dependencies.
How Does GPR Work?
GPR operates by constructing a Gaussian process model that represents the distribution of possible functions that could describe the relationship between the input variables (such as historical stock prices, economic indicators, or company fundamentals) and the target variable (e.g., future stock price or portfolio return). The model is trained on a subset of the available data, known as the training set. During this process, GPR learns the underlying patterns and uncertainties in the data, allowing it to make predictions for new, unseen inputs. Additionally, GPR provides a measure of uncertainty associated with each prediction, enabling investors to quantify the confidence in their forecasts.
Examples of GPR in Investment Applications
Stock Price Forecasting: GPR can be applied to historical stock price data, along with relevant features such as trading volume, company financials, and market indicators, to predict future stock prices. This can aid investors in making informed buy or sell decisions and optimizing their portfolios. Example: Suppose an investor wants to forecast the stock price of a technology company for the next quarter. GPR can be trained on historical stock prices, earnings reports, industry trends, and other relevant factors to generate predictions and associated uncertainty estimates.
Portfolio Optimization: GPR can be used to model the expected returns and risks of different assets or portfolios, considering factors like historical performance, economic conditions, and market correlations. This information can guide investors in constructing well-diversified portfolios that align with their risk tolerance and investment objectives. Example: An investor can employ GPR to predict the expected returns and volatilities of various asset classes (stocks, bonds, commodities, etc.) based on historical data and macroeconomic indicators. These predictions can then be used in portfolio optimization algorithms to determine the optimal asset allocation that maximizes returns while minimizing risk.
Derivative Pricing: GPR can be applied to pricing financial derivatives, such as options and futures contracts, by modeling the underlying asset's price dynamics and accounting for factors like volatility, interest rates, and time to expiration. Example: Consider an investor interested in pricing an option contract on a particular stock. GPR can be trained on historical stock prices, implied volatilities, and other relevant variables to predict the option's fair value and associated uncertainty, enabling informed trading decisions.
Advantages of GPR for Investors
Flexibility: GPR can capture complex, non-linear relationships without imposing restrictive assumptions on the data.
Uncertainty Quantification: GPR provides a measure of uncertainty associated with each prediction, allowing investors to assess the reliability of their forecasts.
Interpretability: GPR models can be interpreted and analyzed to gain insights into the underlying relationships between input variables and the target variable.
Scalability: GPR can handle high-dimensional data and large datasets, making it suitable for diverse investment applications.
Challenges and Limitations
While GPR offers numerous advantages, it's important for investors to be aware of its potential challenges and limitations:
Data Quality: Like any machine learning model, the performance of GPR heavily relies on the quality and representativeness of the training data. Noisy or incomplete data can lead to inaccurate predictions and unreliable uncertainty estimates.
Computational Complexity: GPR can be computationally intensive, especially for large datasets or high-dimensional input spaces. This can make real-time predictions or frequent model updates challenging, depending on the available computational resources.
Hyperparameter Tuning: GPR models have several hyperparameters, such as the kernel function and its parameters, that need to be carefully tuned to achieve optimal performance. This process can be time-consuming and may require domain expertise or advanced optimization techniques.
Interpretability Trade-off: While GPR models can provide insights into the relationships between input variables and the target variable, their interpretability can diminish as the complexity of the input space increases, making it harder to explain the model's predictions.
Gaussian Process Regression (GPR) is a powerful machine learning tool that offers investors a flexible and data-driven approach to forecasting and decision-making. By capturing complex patterns in financial data and providing uncertainty estimates, GPR can aid in stock price predictions, portfolio optimization, derivative pricing, and various other investment applications. As the financial industry continues to embrace data-driven methodologies, GPR is poised to become an increasingly valuable asset in an investor's analytical toolkit.
Comentários